Subseqüência crescente mais longa | DP-3
Já discutimos Subproblemas de sobreposição e propriedades de subestruturas ótimas .
Agora, vamos discutir o problema da Subseqüência mais longa (LIS) como um exemplo de problema que pode ser resolvido usando a Programação Dinâmica.
O problema da Subseqüência mais longa (LIS) é encontrar o comprimento da subseqüência mais longa de uma determinada seqüência de forma que todos os elementos da subsequência sejam classificados em ordem crescente. Por exemplo, o comprimento de LIS para {10, 22, 9, 33, 21, 50, 41, 60, 80} é 6 e LIS é {10, 22, 33, 50, 60, 80}.
Exemplos:
Input: arr[] = {3, 10, 2, 1, 20} Output: Length of LIS = 3 The longest increasing subsequence is 3, 10, 20 Input: arr[] = {3, 2} Output: Length of LIS = 1 The longest increasing subsequences are {3} and {2} Input: arr[] = {50, 3, 10, 7, 40, 80} Output: Length of LIS = 4 The longest increasing subsequence is {3, 7, 40, 80}
Método 1 : Recursão. Subestrutura
ótima: Seja arr [0..n-1] a array de entrada e L (i) o comprimento do LIS terminando no índice i, de modo que arr [i] seja o último elemento do LIS.
Então, L (i) pode ser escrito recursivamente como:
L(i) = 1 + max( L(j) ) where 0 < j < i and arr[j] < arr[i]; or L(i) = 1, if no such j exists.
Para encontrar o LIS para um determinado array, precisamos retornar max (L (i)) onde 0 <i <n.
Formalmente, o comprimento da subsequência crescente mais longa terminando no índice i, será 1 maior que o máximo de comprimentos de todas as subsequências crescentes mais longas terminando nos índices antes de i, onde arr [j] <arr [i] (j <i).
Assim, vemos que o problema LIS satisfaz a propriedade de subestrutura ótima, pois o problema principal pode ser resolvido usando soluções para subproblemas.
A árvore recursiva fornecida abaixo tornará a abordagem mais clara:
Input : arr[] = {3, 10, 2, 11} f(i): Denotes LIS of subarray ending at index 'i' (LIS(1)=1) f(4) {f(4) = 1 + max(f(1), f(2), f(3))} / | \ f(1) f(2) f(3) {f(3) = 1, f(2) and f(1) are > f(3)} | | \ f(1) f(2) f(1) {f(2) = 1 + max(f(1)} | f(1) {f(1) = 1}
Abaixo está a implementação da abordagem recursiva:
/* A Naive C++ recursive implementation
of LIS problem */
#include <iostream>
using namespace std;
/* To make use of recursive calls, this
function must return two things:
1) Length of LIS ending with element arr[n-1].
We use max_ending_here for this purpose
2) Overall maximum as the LIS may end with
an element before arr[n-1] max_ref is
used this purpose.
The value of LIS of full array of size n
is stored in *max_ref which is our final result
*/
int _lis(int arr[], int n, int* max_ref)
{
/* Base case */
if (n == 1)
return 1;
// 'max_ending_here' is length of LIS
// ending with arr[n-1]
int res, max_ending_here = 1;
/* Recursively get all LIS ending with arr[0],
arr[1] ... arr[n-2]. If arr[i-1] is smaller
than arr[n-1], and max ending with arr[n-1]
needs to be updated, then update it */
for (int i = 1; i < n; i++) {
res = _lis(arr, i, max_ref);
if (arr[i - 1] < arr[n - 1]
&& res + 1 > max_ending_here)
max_ending_here = res + 1;
}
// Compare max_ending_here with the overall
// max. And update the overall max if needed
if (*max_ref < max_ending_here)
*max_ref = max_ending_here;
// Return length of LIS ending with arr[n-1]
return max_ending_here;
}
// The wrapper function for _lis()
int lis(int arr[], int n)
{
// The max variable holds the result
int max = 1;
// The function _lis() stores its result in max
_lis(arr, n, &max);
// returns max
return max;
}
/* Driver program to test above function */
int main()
{
int arr[] = { 10, 22, 9, 33, 21, 50, 41, 60 };
int n = sizeof(arr) / sizeof(arr[0]);
cout <<"Length of lis is "<< lis(arr, n);
return 0;
}
// This code is contributed by shivanisinghss2110
/* A Naive C recursive implementation
of LIS problem */
#include <stdio.h>
#include <stdlib.h>
/* To make use of recursive calls, this
function must return two things:
1) Length of LIS ending with element arr[n-1].
We use max_ending_here for this purpose
2) Overall maximum as the LIS may end with
an element before arr[n-1] max_ref is
used this purpose.
The value of LIS of full array of size n
is stored in *max_ref which is our final result
*/
int _lis(int arr[], int n, int* max_ref)
{
/* Base case */
if (n == 1)
return 1;
// 'max_ending_here' is length of LIS
// ending with arr[n-1]
int res, max_ending_here = 1;
/* Recursively get all LIS ending with arr[0],
arr[1] ... arr[n-2]. If arr[i-1] is smaller
than arr[n-1], and max ending with arr[n-1]
needs to be updated, then update it */
for (int i = 1; i < n; i++) {
res = _lis(arr, i, max_ref);
if (arr[i - 1] < arr[n - 1]
&& res + 1 > max_ending_here)
max_ending_here = res + 1;
}
// Compare max_ending_here with the overall
// max. And update the overall max if needed
if (*max_ref < max_ending_here)
*max_ref = max_ending_here;
// Return length of LIS ending with arr[n-1]
return max_ending_here;
}
// The wrapper function for _lis()
int lis(int arr[], int n)
{
// The max variable holds the result
int max = 1;
// The function _lis() stores its result in max
_lis(arr, n, &max);
// returns max
return max;
}
/* Driver program to test above function */
int main()
{
int arr[] = { 10, 22, 9, 33, 21, 50, 41, 60 };
int n = sizeof(arr) / sizeof(arr[0]);
printf("Length of lis is %d", lis(arr, n));
return 0;
}
/* A Naive Java Program for LIS Implementation */
class LIS {
static int max_ref; // stores the LIS
/* To make use of recursive calls, this function must
return two things: 1) Length of LIS ending with element
arr[n-1]. We use max_ending_here for this purpose 2)
Overall maximum as the LIS may end with an element
before arr[n-1] max_ref is used this purpose.
The value of LIS of full array of size n is stored in
*max_ref which is our final result */
static int _lis(int arr[], int n)
{
// base case
if (n == 1)
return 1;
// 'max_ending_here' is length of LIS ending with
// arr[n-1]
int res, max_ending_here = 1;
/* Recursively get all LIS ending with arr[0],
arr[1] ... arr[n-2]. If arr[i-1] is smaller
than arr[n-1], and max ending with arr[n-1] needs
to be updated, then update it */
for (int i = 1; i < n; i++) {
res = _lis(arr, i);
if (arr[i - 1] < arr[n - 1]
&& res + 1 > max_ending_here)
max_ending_here = res + 1;
}
// Compare max_ending_here with the overall max. And
// update the overall max if needed
if (max_ref < max_ending_here)
max_ref = max_ending_here;
// Return length of LIS ending with arr[n-1]
return max_ending_here;
}
// The wrapper function for _lis()
static int lis(int arr[], int n)
{
// The max variable holds the result
max_ref = 1;
// The function _lis() stores its result in max
_lis(arr, n);
// returns max
return max_ref;
}
// driver program to test above functions
public static void main(String args[])
{
int arr[] = { 10, 22, 9, 33, 21, 50, 41, 60 };
int n = arr.length;
System.out.println("Length of lis is " + lis(arr, n)
+ "\n");
}
}
/*This code is contributed by Rajat Mishra*/
# A naive Python implementation of LIS problem
""" To make use of recursive calls, this function must return
two things:
1) Length of LIS ending with element arr[n-1]. We use
max_ending_here for this purpose
2) Overall maximum as the LIS may end with an element
before arr[n-1] max_ref is used this purpose.
The value of LIS of full array of size n is stored in
*max_ref which is our final result """
# global variable to store the maximum
global maximum
def _lis(arr, n):
# to allow the access of global variable
global maximum
# Base Case
if n == 1:
return 1
# maxEndingHere is the length of LIS ending with arr[n-1]
maxEndingHere = 1
"""Recursively get all LIS ending with arr[0], arr[1]..arr[n-2]
IF arr[n-1] is maller than arr[n-1], and max ending with
arr[n-1] needs to be updated, then update it"""
for i in xrange(1, n):
res = _lis(arr, i)
if arr[i-1] < arr[n-1] and res+1 > maxEndingHere:
maxEndingHere = res + 1
# Compare maxEndingHere with overall maximum. And
# update the overall maximum if needed
maximum = max(maximum, maxEndingHere)
return maxEndingHere
def lis(arr):
# to allow the access of global variable
global maximum
# length of arr
n = len(arr)
# maximum variable holds the result
maximum = 1
# The function _lis() stores its result in maximum
_lis(arr, n)
return maximum
# Driver program to test the above function
arr = [10, 22, 9, 33, 21, 50, 41, 60]
n = len(arr)
print "Length of lis is ", lis(arr)
# This code is contributed by NIKHIL KUMAR SINGH
using System;
/* A Naive C# Program for LIS Implementation */
class LIS {
static int max_ref; // stores the LIS
/* To make use of recursive calls, this function must
return two things: 1) Length of LIS ending with element
arr[n-1]. We use max_ending_here for this purpose 2)
Overall maximum as the LIS may end with an element
before arr[n-1] max_ref is used this purpose.
The value of LIS of full array of size n is stored in
*max_ref which is our final result */
static int _lis(int[] arr, int n)
{
// base case
if (n == 1)
return 1;
// 'max_ending_here' is length of LIS ending with
// arr[n-1]
int res, max_ending_here = 1;
/* Recursively get all LIS ending with arr[0],
arr[1] ... arr[n-2]. If arr[i-1] is smaller
than arr[n-1], and max ending with arr[n-1] needs
to be updated, then update it */
for (int i = 1; i < n; i++) {
res = _lis(arr, i);
if (arr[i - 1] < arr[n - 1]
&& res + 1 > max_ending_here)
max_ending_here = res + 1;
}
// Compare max_ending_here with the overall max. And
// update the overall max if needed
if (max_ref < max_ending_here)
max_ref = max_ending_here;
// Return length of LIS ending with arr[n-1]
return max_ending_here;
}
// The wrapper function for _lis()
static int lis(int[] arr, int n)
{
// The max variable holds the result
max_ref = 1;
// The function _lis() stores its result in max
_lis(arr, n);
// returns max
return max_ref;
}
// driver program to test above functions
public static void Main()
{
int[] arr = { 10, 22, 9, 33, 21, 50, 41, 60 };
int n = arr.Length;
Console.Write("Length of lis is " + lis(arr, n)
+ "\n");
}
}
<script>
/* A Naive javascript Program for LIS Implementation */
let max_ref; // stores the LIS
/* To make use of recursive calls, this function must return
two things:
1) Length of LIS ending with element arr[n-1]. We use
max_ending_here for this purpose
2) Overall maximum as the LIS may end with an element
before arr[n-1] max_ref is used this purpose.
The value of LIS of full array of size n is stored in
*max_ref which is our final result */
function _lis(arr,n)
{
// base case
if (n == 1)
return 1;
// 'max_ending_here' is length of LIS ending with arr[n-1]
let res, max_ending_here = 1;
/* Recursively get all LIS ending with arr[0], arr[1] ...
arr[n-2]. If arr[i-1] is smaller than arr[n-1], and
max ending with arr[n-1] needs to be updated, then
update it */
for (let i = 1; i < n; i++)
{
res = _lis(arr, i);
if (arr[i-1] < arr[n-1] && res + 1 > max_ending_here)
max_ending_here = res + 1;
}
// Compare max_ending_here with the overall max. And
// update the overall max if needed
if (max_ref < max_ending_here)
max_ref = max_ending_here;
// Return length of LIS ending with arr[n-1]
return max_ending_here;
}
// The wrapper function for _lis()
function lis(arr,n)
{
// The max variable holds the result
max_ref = 1;
// The function _lis() stores its result in max
_lis( arr, n);
// returns max
return max_ref;
}
// driver program to test above functions
let arr=[10, 22, 9, 33, 21, 50, 41, 60 ]
let n = arr.length;
document.write("Length of lis is "
+ lis(arr, n) + "<br>");
// This code is contributed by avanitrachhadiya2155
</script>
Saída:
Length of lis is 5
Análise de complexidade:
- Complexidade de tempo: a complexidade de tempo dessa abordagem recursiva é exponencial, pois há um caso de subproblemas sobrepostos, conforme explicado no diagrama de árvore recursiva acima.
- Espaço auxiliar: O (1). Nenhum espaço externo usado para armazenar valores além do espaço interno da pilha.
Método 2 : Programação dinâmica.
Podemos ver que existem muitos subproblemas na solução recursiva acima que são resolvidos repetidamente. Portanto, esse problema tem a propriedade Overlapping Substructure e a recomputação dos mesmos subproblemas pode ser evitada usando Memoização ou Tabulação.
A simulação da abordagem deixará as coisas claras:
Input : arr[] = {3, 10, 2, 11} LIS[] = {1, 1, 1, 1} (initially)
Simulação de iteração:
- arr [2]> arr [1] {LIS [2] = máx (LIS [2], LIS [1] +1) = 2}
- arr [3] <arr [1] {Sem alteração}
- arr [3] <arr [2] {Sem alteração}
- arr [4]> arr [1] {LIS [4] = máx (LIS [4], LIS [1] +1) = 2}
- arr [4]> arr [2] {LIS [4] = máx (LIS [4], LIS [2] +1) = 3}
- arr [4]> arr [3] {LIS [4] = máx (LIS [4], LIS [3] +1) = 3}
Podemos evitar a recomputação de subproblemas usando a tabulação conforme mostrado no código a seguir:
Abaixo está a implementação da abordagem acima:
/* Dynamic Programming C++ implementation
of LIS problem */
#include <bits/stdc++.h>
using namespace std;
/* lis() returns the length of the longest
increasing subsequence in arr[] of size n */
int lis(int arr[], int n)
{
int lis[n];
lis[0] = 1;
/* Compute optimized LIS values in
bottom up manner */
for (int i = 1; i < n; i++) {
lis[i] = 1;
for (int j = 0; j < i; j++)
if (arr[i] > arr[j] && lis[i] < lis[j] + 1)
lis[i] = lis[j] + 1;
}
// Return maximum value in lis[]
return *max_element(lis, lis + n);
}
/* Driver program to test above function */
int main()
{
int arr[] = { 10, 22, 9, 33, 21, 50, 41, 60 };
int n = sizeof(arr) / sizeof(arr[0]);
printf("Length of lis is %d\n", lis(arr, n));
return 0;
}
/* Dynamic Programming Java implementation
of LIS problem */
class LIS {
/* lis() returns the length of the longest
increasing subsequence in arr[] of size n */
static int lis(int arr[], int n)
{
int lis[] = new int[n];
int i, j, max = 0;
/* Initialize LIS values for all indexes */
for (i = 0; i < n; i++)
lis[i] = 1;
/* Compute optimized LIS values in
bottom up manner */
for (i = 1; i < n; i++)
for (j = 0; j < i; j++)
if (arr[i] > arr[j] && lis[i] < lis[j] + 1)
lis[i] = lis[j] + 1;
/* Pick maximum of all LIS values */
for (i = 0; i < n; i++)
if (max < lis[i])
max = lis[i];
return max;
}
public static void main(String args[])
{
int arr[] = { 10, 22, 9, 33, 21, 50, 41, 60 };
int n = arr.length;
System.out.println("Length of lis is " + lis(arr, n)
+ "\n");
}
}
/*This code is contributed by Rajat Mishra*/
# Dynamic programming Python implementation
# of LIS problem
# lis returns length of the longest
# increasing subsequence in arr of size n
def lis(arr):
n = len(arr)
# Declare the list (array) for LIS and
# initialize LIS values for all indexes
lis = [1]*n
# Compute optimized LIS values in bottom up manner
for i in range(1, n):
for j in range(0, i):
if arr[i] > arr[j] and lis[i] < lis[j] + 1:
lis[i] = lis[j]+1
# Initialize maximum to 0 to get
# the maximum of all LIS
maximum = 0
# Pick maximum of all LIS values
for i in range(n):
maximum = max(maximum, lis[i])
return maximum
# end of lis function
# Driver program to test above function
arr = [10, 22, 9, 33, 21, 50, 41, 60]
print "Length of lis is", lis(arr)
# This code is contributed by Nikhil Kumar Singh
/* Dynamic Programming C# implementation of LIS problem */
using System;
class LIS {
/* lis() returns the length of the longest increasing
subsequence in arr[] of size n */
static int lis(int[] arr, int n)
{
int[] lis = new int[n];
int i, j, max = 0;
/* Initialize LIS values for all indexes */
for (i = 0; i < n; i++)
lis[i] = 1;
/* Compute optimized LIS values in bottom up manner
*/
for (i = 1; i < n; i++)
for (j = 0; j < i; j++)
if (arr[i] > arr[j] && lis[i] < lis[j] + 1)
lis[i] = lis[j] + 1;
/* Pick maximum of all LIS values */
for (i = 0; i < n; i++)
if (max < lis[i])
max = lis[i];
return max;
}
public static void Main()
{
int[] arr = { 10, 22, 9, 33, 21, 50, 41, 60 };
int n = arr.Length;
Console.WriteLine("Length of lis is " + lis(arr, n)
+ "\n");
}
// This code is contributed by Ryuga
}
<script>
// Dynamic Programming Javascript implementation
// of LIS problem
// lis() returns the length of the longest
// increasing subsequence in arr[] of size n
function lis(arr, n)
{
let lis = Array(n).fill(0);
let i, j, max = 0;
// Initialize LIS values for all indexes
for(i = 0; i < n; i++)
lis[i] = 1;
// Compute optimized LIS values in
// bottom up manner
for(i = 1; i < n; i++)
for(j = 0; j < i; j++)
if (arr[i] > arr[j] && lis[i] < lis[j] + 1)
lis[i] = lis[j] + 1;
// Pick maximum of all LIS values
for(i = 0; i < n; i++)
if (max < lis[i])
max = lis[i];
return max;
}
// Driver code
let arr = [ 10, 22, 9, 33, 21, 50, 41, 60 ];
let n = arr.length;
document.write("Length of lis is " + lis(arr, n) + "\n");
// This code is contributed by avijitmondal1998
</script>
O comprimento de lis é 5
Análise de complexidade:
- Complexidade de tempo: O (n 2 ).
Como o loop aninhado é usado. - Espaço auxiliar: O (n).
Uso de qualquer array para armazenar valores LIS em cada índice.
Nota: A complexidade de tempo da solução de Programação Dinâmica (DP) acima é O (n ^ 2) e há uma solução O (N log N) para o problema LIS. Não discutimos a solução O (N log N) aqui, pois o objetivo deste artigo é explicar a Programação Dinâmica com um exemplo simples. Veja a postagem abaixo para a solução O (N log N).
Maior tamanho de subseqüência crescente (N log N)
Método 3: Programação Dinâmica
Se observarmos de perto o problema, podemos convertê-lo no mais longo Problema Subseqüente Comum. Em primeiro lugar, iremos criar outro array de elementos únicos do array original e classificá-lo. Agora, a maior subseqüência crescente de nosso array deve estar presente como uma subsequência em nosso array ordenado. É por isso que nosso problema agora se reduz a encontrar a subsequência comum entre as duas arrayes.
Eg. arr =[50,3,10,7,40,80] // Sorted array arr1 = [3,7,10,40,50,80] // LIS is longest common subsequence between the two arrays ans = 4 The longest increasing subsequence is {3, 7, 40, 80}
# Dynamic Programming Approach of Finding LIS by reducing the problem to longest common Subsequence
def lis(a):
n=len(a)
# Creating the sorted list
b=sorted(list(set(a)))
m=len(b)
# Creating dp table for storing the answers of sub problems
dp=[[-1 for i in range(m+1)] for j in range(n+1)]
# Finding Longest common Subsequence of the two arrays
for i in range(n+1):
for j in range(m+1):
if i==0 or j==0:
dp[i][j]=0
elif a[i-1]==b[j-1]:
dp[i][j]=1+dp[i-1][j-1]
else:
dp[i][j]=max(dp[i-1][j],dp[i][j-1])
return dp[-1][-1]
# Driver program to test above function
arr = [10, 22, 9, 33, 21, 50, 41, 60]
print("Length of lis is ", lis(arr))
# This code is Contributed by Dheeraj Khatri
O comprimento de lis é 5
Análise de complexidade : O (n * n)
Como o loop aninhado é usado
Complexidade do espaço : O (n * n)
Como uma array é usada para armazenar os valores.
https://www.youtube.com/watch?v=Ns4LCeeOFS4
Escreva comentários se encontrar algo incorreto ou se quiser compartilhar mais informações sobre o tópico discutido acima.
As postagens do blog Acervo Lima te ajudaram? Nos ajude a manter o blog no ar!
Faça uma doação para manter o blog funcionando.
70% das doações são no valor de R$ 5,00...
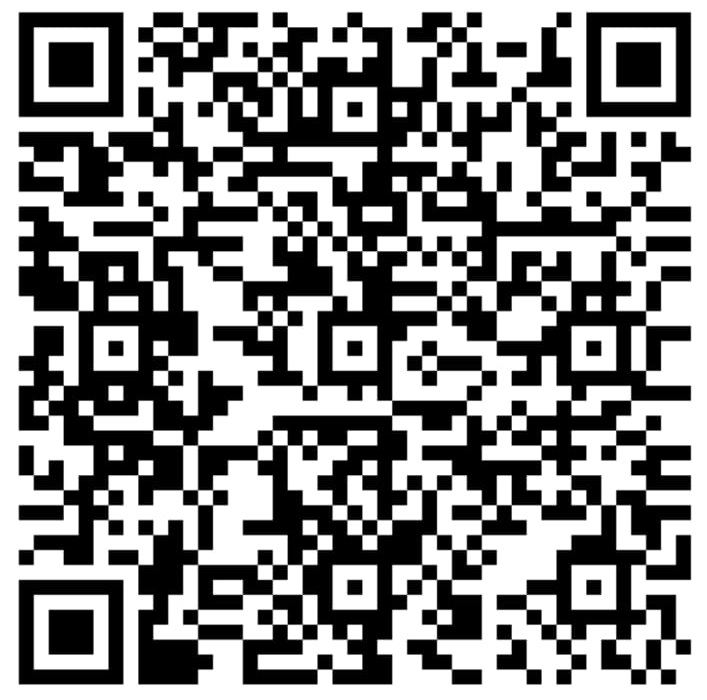
Diógenes Lima da Silva
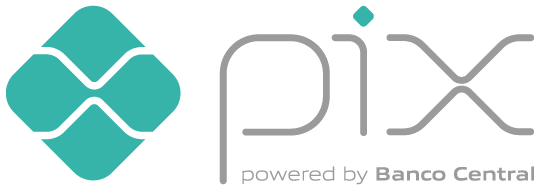